From Google to Generative AI: Are LLMs Taking Over The Internet?
The world wide web as we know it is currently in the throes of profound transformation. At the epicenter of this shift we find Large Language Models (LLMs), which are a form of artificial intelligence that is capable of understanding as well as generating human-like language. The applications of LLMs range from chatbots to creative writers, but their true potential is to be found in how they are reshaping the very structure of the digital environment we know.
As far as we’ve known the web, its experiences have revolved around static interfaces, manual content management and keyword-based search, but now, LLMs are upending this paradigm. As they interpret context, intent and meaning, they enable websites to become more adaptable, personalized and intelligent. The way in which businesses manage their content, organize their assets or optimize user experiences is bound to rapidly evolve in light of these changes.
In this article we’ll explore how LLMs are currently influencing the evolution of the web, particularly in the realm of content-heavy domains. We’ll see the intersection of these models with other elements such as content management through DAMs or web optimization. How can companies streamline internal workflows, improve asset discovery and enhance digital experiences in the face of a rapidly-changing web environment? We know businesses are navigating an increasingly complex digital ecosystem, and understanding the impact of LLMs is now part of the key learnings for those seeking success.
What are LLMs and why do they matter?
As we’ve briefly seen, LLMs are a type of artificial intelligence that is trained on vast numbers of text data. They are models such as OpenAI’s GPT, or Google’s Gemini. They learn to understand as well as generate human language through identifying patterns across billions of words. What sets them apart? Their incredible ability to process not just the words themselves, but the context and the intent behind them. This enables them to answer questions, summarize content, draft text, translate languages and much more.
LLMs are much more than just advanced, fancy text generators. Their real value is in how they are currently transforming the way we interact with digital information. Users don’t need to rely on exact keywords anymore, they can communicate naturally with the systems and receive intelligent, relevant and tailored results. This entails a major shift from traditional computing, where information retrieval and consumption have often been rigid and unintuitive.
What does this imply for businesses? Well, it means that LLMs can automate labor-intensive tasks like content tagging, document classification and knowledge management. They can also be the driving force behind smarter internal search tools, simplify navigation in internal digital asset libraries and even support creative processes through the generation of content ideas, spell checking or documentation.
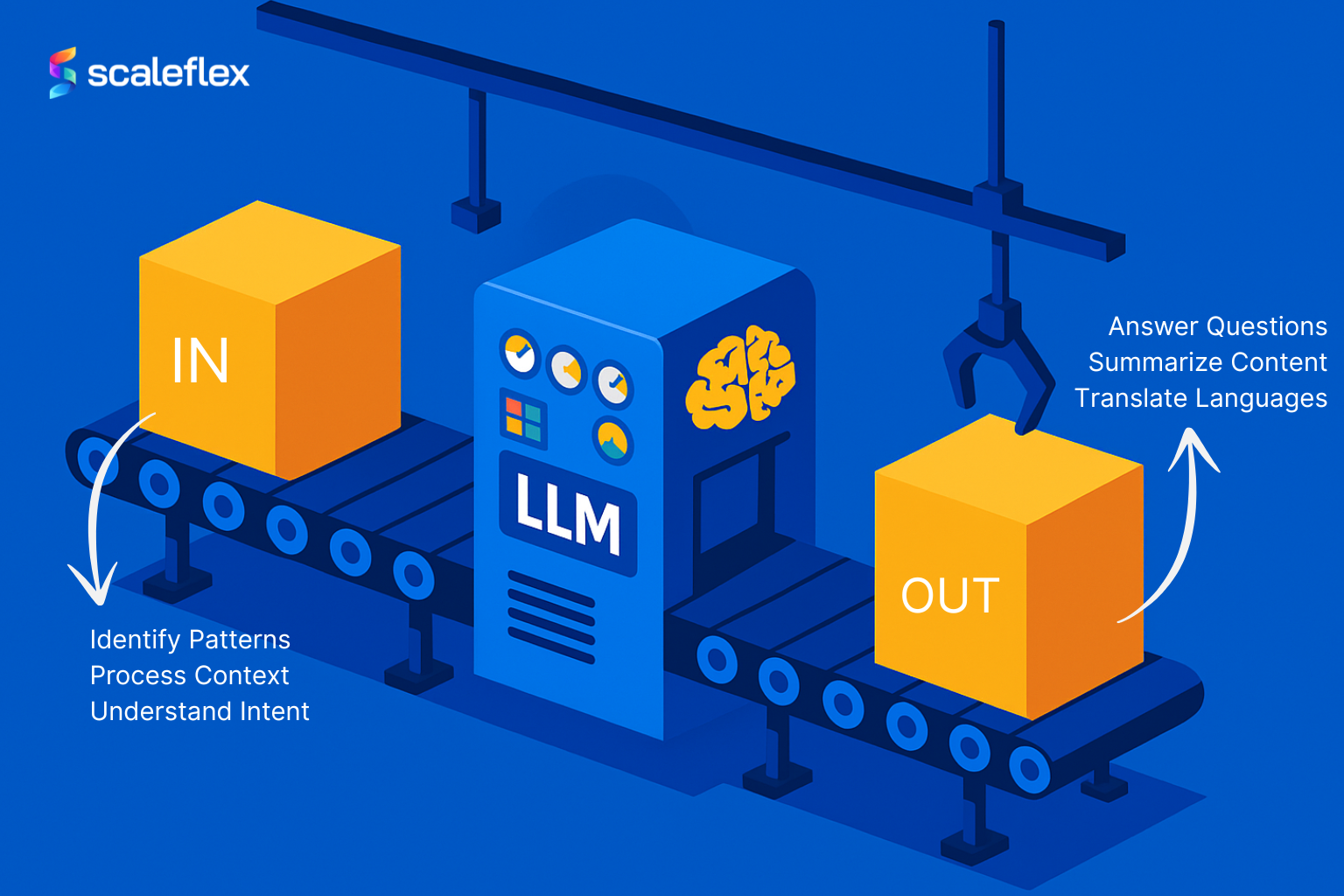
Companies continue to grow and amass visual and textual data. LLMS can offer a scalable and intelligent way to manage and extract valuable information from them. Integrating them into existing tools or content ecosystems could reshape digital workflows and set the stage for an intuitive and responsive web environment.
From search to understanding: How LLMs are changing online discovery
The most immediately evident way in which LLMs are reshaping the web environment is in how we search for and discover content. Traditionally, search engines as well as internal websites have relied on exact keyword matches, and this system will typically forego nuance, context or user intent. It’s an approach that can lead to frustration, especially when users don’t know the exact terminology or have a hard time applying the information to their particular needs or situation.
How are LLMs changing the rules? By enabling semantic search, there’s no longer a need to literally match words, as they can interpret the meaning behind every query. This enables users to ask questions in a nonchalant manner and still receive accurate, personalized, context-aware results. This is a considerable breakthrough for content-heavy businesses. When they’re integrated into their digital asset management systems, LLMs make it easier to retrieve the right content, even when dealing with vast, complex libraries. The language model is able to “read” the captions, tags, metadata and even textual content associated with each asset, and then trace it back to the user query with uncanny precision.
In a nutshell: discovery is more intuitive, efficient and aligned with the way in which humans naturally think and communicate. This paradigm shift will save time, but not only, as it will also improve work quality and decision-making abilities.
Smart asset management with LLMs
Businesses continue to scale, and so do their digital libraries, sometimes to the point where managing, organizing and retrieving content becomes a major operational challenge. Companies that employ advanced DAM systems may reap the benefits of early LLM implementation, as DAM providers introduce this new level of intelligence to the process.
As we know, assets have traditionally been manually tagged and categorized. This is a time-consuming and inconsistent task that is prone to error when left in the hands of weary employees. LLMs automate much of this work as they analyze the content and context of every single file, generate relevant descriptions, metadata and even suggest usages. LLMs can read any accompanying text, recognize patterns and make connections that would take any human far too long to identify.
LLMs also make asset libraries more searchable thanks to natural language queries. Users can, instead of relying on the exact file name or tag, describe what they’re looking for, for instance “logo variations from last spring’s campaign” or “images suitable for an eco-friendly product launch” and get precise and pertinent results. Pretty amazing. This change of paradigm goes way beyond time savings, as it improves collaboration across teams, particularly when working across geographies or departments.
Visual AI meets LLMs: a new frontier in content intelligence
What would happen if LLMs and Visual AI were to converge? LLMS excel at interpreting and generating language, while Visual AI has the ability to analyze, classify and understand images and videos. Together they’d create a rather powerful hybrid tool, capable of delivering more content intelligence than ever before.
In practical terms, businesses could analyze both the visual and textual components of their digital assets, in their context. It would be a system that would not only recognize the beach contained in the photo, but also understand that this means the visual is associated to a summer campaign, linked to specific product messaging, and could then retrieve it or repurpose it at anyone’s simple request.
This synergy could enhance everything, ranging from search to personalization. Teams would be able to type “assets that feel calm and minimal for a wellness launch”, and retrieve pertinent content that matches the tone, even when those precise words couldn’t be found in the tags. This would reduce asset waste, accelerate creative workflows and ensure brand coherence across all content touchpoints.
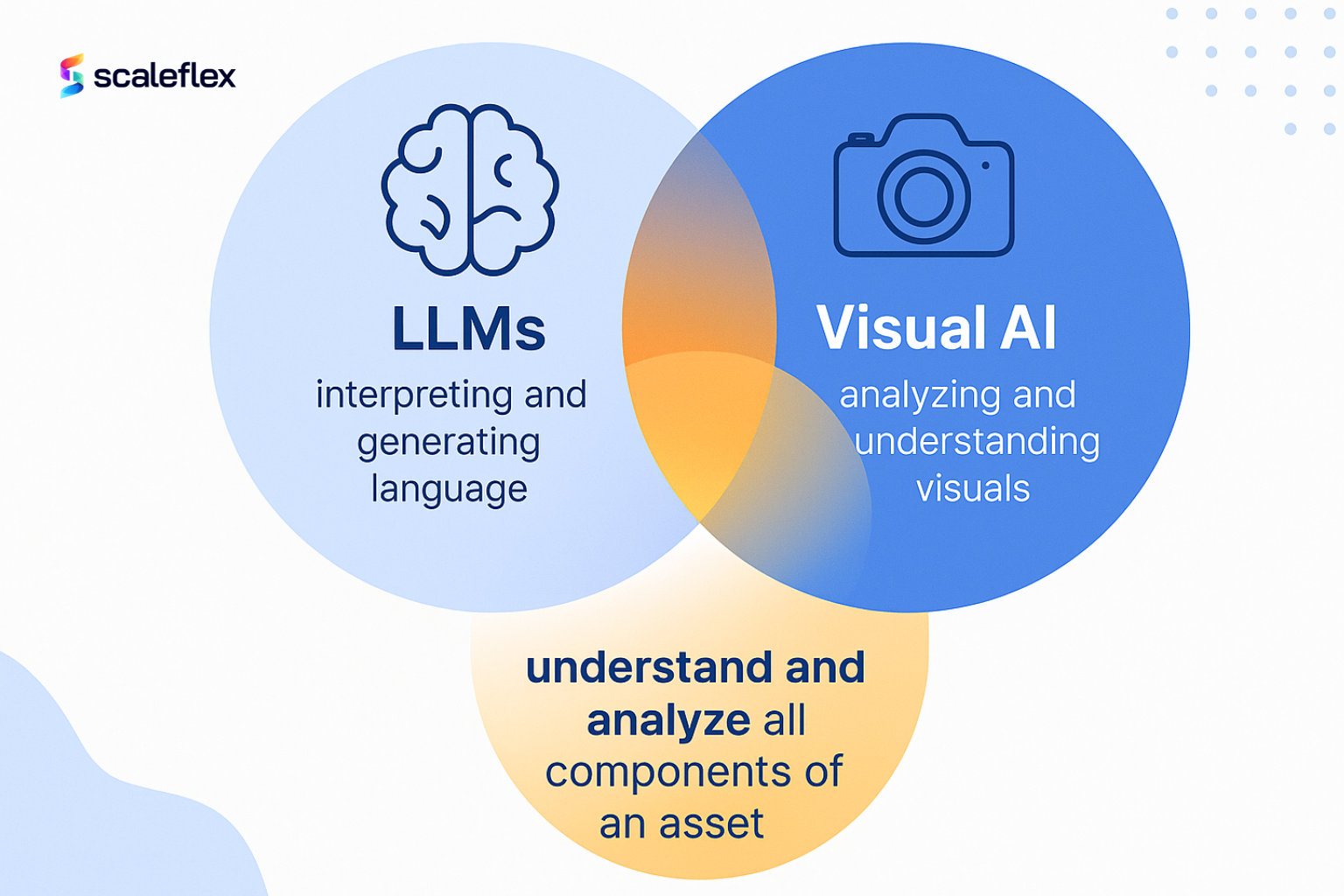
Personalization and web optimization at scale
Personalization is a client expectation in our current digital landscape. Every user wants to find content that speaks to his needs, his interests and his particular context. That’s where LLMs play a special role, as they make tailored experiences possible at any scale.
LLMs can analyze user behaviour, preferences and engagement patterns to then generate content that will feel individualized. Be it recommending the right product visuals, or tailoring landing page text, or guiding users through relevant website journeys, these models can help businesses speak the language of each visitor without the need to manually segment their audiences or create endless variations.
This type of intelligent personalization is particularly valuable in the areas of marketing and e-commerce, as these teams work with vast asset libraries. For example, a Visual AI system that is supported by LLMs can adapt imagery shown on a homepage depending on the user’s location, his previous browsing history or current seasonal trends, all to ensure that what the user sees will resonate with him, in real time.
LLMs serve to, ultimately, smooth out the friction between user intent and brand communication. They help brands create a richer, more connected web experience that can feel intuitive, relevant and human, as they turn each touchpoint into a crucial moment that drives engagement and conversion.
Challenges and ethical considerations
LLMs and Visual AI are a powerful combination, but their integration into digital ecosystems can raise concerns and ethical questions. Firstly, there can be issues around data quality and bias. As these models learn from vast datasets, these may reflect existing societal inequalities or cultural blind spots. It is important to properly manage them to avoid skewed outputs, misinterpretations or exclusions.
Another concern can be transparency. When an AI system generates or recommends content, it’s not clear how it comes to its decisions. There’s a “black box” effect that can complicate accountability, particularly when it comes to brand voice, accuracy or fairness.
Lastly, privacy and content ownership are increasingly relevant topics Companies are leaning on generative tools, but in order to do so they must navigate copyright laws, user content and the responsible use of internal data.
To effectively address these concerns will require more than technical solutions - implementing thoughtful governance, diverse training data and commitment to ethical design can help keep human values center stage.
Looking ahead: what does this mean for brands and teams?
The rise of LLMs will shift how we navigate the web, how we create and interact with digital content. For companies and teams, this will mean a move going beyond static workflows, and embracing more adaptive, responsive and intuitive systems. Web navigation and content discovery will become one fluid, natural interaction. In companies, collaboration will become smoother as LLMs and AI help bridge the gaps between creative, marketing and technical roles.
Brands have a unique chance to operate with greater precision than ever before to ensure that the right messages are reaching the right audience, in the right format, at the right time.
In short, LLMs aren’t just flashy new tools. They are currently reshaping the very foundations of our digital experiences, and embracing them mindfully will better equip us for further innovation.